The Ultimate Guide to Data Labeling Tools: Enhancing Your Business Efficiency
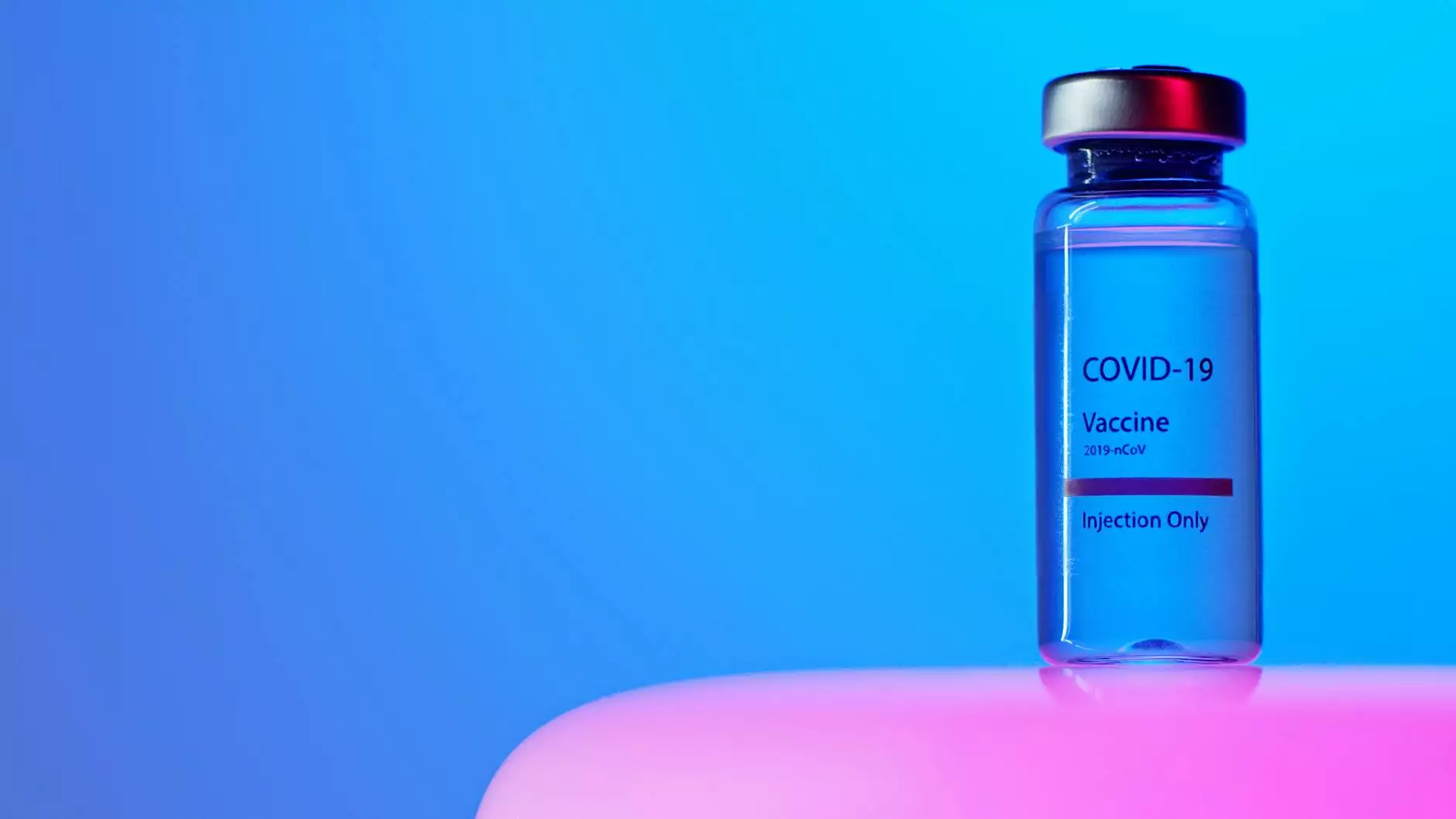
In today's rapidly evolving digital landscape, businesses are increasingly relying on data-driven strategies to make informed decisions. At the heart of these strategies lies the need for accurate and efficient data processing. One critical component of this is the use of a data labeling tool. In this comprehensive guide, we will explore what data labeling tools are, their benefits, and how they can elevate your business operations.
What is a Data Labeling Tool?
A data labeling tool is a software solution designed to add metadata to raw data, categorizing it for easier analysis. In the context of machine learning and artificial intelligence, data labeling is a crucial step that enables models to learn from labeled datasets and make predictions or decisions based on new, unseen data.
Importance of Data Labeling
Effective data labeling serves multiple purposes:
- Training Machine Learning Models: Labeled data serves as the foundation for training models. Without it, models cannot learn to differentiate between classes.
- Improving Accuracy: High-quality labeled datasets lead to better model performance, which ultimately enhances business outcomes.
- Streamlining Decision-Making: By automating data labeling, businesses can accelerate analytics and improve decision-making processes significantly.
Types of Data Annotation Platforms
Data annotation platforms come in various forms, catering to different needs based on the data types and complexities involved:
1. Image and Video Annotation Platforms
These platforms are specialized for visual data. Businesses in sectors like retail, automotive, and healthcare often require:
- Bounding Box Annotation: This method involves drawing boxes around objects in images or frames in videos for object detection tasks.
- Semantic Segmentation: Distinguishing between different segments of an image for more granular understanding.
- Transcription Services: Converting spoken language into text in video annotations, useful for training speech recognition models.
2. Text Annotation Platforms
Text annotation is essential in understanding sentiments, identifying named entities, and categorizing documents. Key techniques include:
- Named Entity Recognition (NER): Identifying proper nouns and categorizing them as people, organizations, or locations.
- Sentiment Analysis: Classifying text based on sentiment, which is vital for marketing and customer feedback insights.
- Topic Tagging: Assigning tags to text data to facilitate categorization and searchability.
How to Choose the Right Data Labeling Tool
Selecting a suitable data labeling tool is crucial for efficiency and quality in your operations. Consider the following factors:
1. Scalability
Choose tools that can grow with your business needs. A scalable platform can handle massive volumes of data without compromising performance.
2. Integration Capabilities
If your current systems can easily integrate with the labeling tool, you will streamline workflow and data management.
3. Usability
A user-friendly interface ensures that your team can quickly adapt and start generating labeled data without significant training.
Benefits of Implementing Data Labeling Tools
Integrating a data labeling tool into your business model offers several advantages:
1. Improved Efficiency
Automation in data labeling reduces manual effort, allowing your team to focus on higher-value tasks and reducing the likelihood of human error.
2. Enhanced Data Accuracy
Professional grade data labeling tools often come equipped with quality assurance processes that enhance the overall accuracy of your datasets.
3. Cost-Effectiveness
Investing in efficient data labeling solutions can significantly lower costs associated with data processing over time, making them a financially sound choice.
Case Studies: Success Stories of Data Labeling in Business
To illustrate the impact of data labeling tools, let's examine a few case studies:
Case Study 1: Retail Analytics
A leading retail company utilized a data labeling platform to categorize customer feedback from various channels. By employing text analysis and sentiment categorization, they gained insights that drove a 30% increase in customer satisfaction scores within one quarter.
Case Study 2: Autonomous Vehicles
An automotive manufacturer developed its autonomous vehicle technology by leveraging image annotation and spatial understanding. Using advanced data labeling tools, they significantly improved the accuracy of object detection, which is crucial for the safety of self-driving cars.
Future of Data Labeling Tools
The future of data labeling tools lies in automation and machine learning. As these technologies advance, we can expect:
- Automated Labeling Processes: Tools that can learn from existing labeled datasets and apply this knowledge to new data streams.
- AI-Powered Quality Control: Utilizing AI to check the quality of labeled data in real-time, ensuring accuracy and consistency.
- Collaborative Annotation Environments: Tools that allow for seamless collaboration among teams, enhancing collective efficiency.
Final Thoughts
As we navigate through the era of big data and artificial intelligence, businesses must recognize the immense potential that lies within effective data labeling. By implementing a reliable data labeling tool, you not only improve your analytics and machine learning outcomes but also equip your organization for sustainable growth.
Visit KeyLabs.ai to explore our innovative solutions tailored to meet your data annotation needs. Embrace the future of data with confidence and drive your business to unprecedented heights!